As digital banking becomes the new norm, the risks associated with fraud, credit defaults, and cybersecurity breaches are also evolving. Traditional risk assessment systems are proving insufficient in keeping up with the volume and complexity of modern financial data. That’s when machine learning takes the spotlight, transforming how decisions are made.
By automating pattern recognition and predicting anomalies in real-time, machine learning is not only helping banks detect risks early but also build smarter, more secure digital financial ecosystems.
Why Traditional Risk Models Fall Short
Traditional banking risk models typically rely on static rules and historical data. These models:
- Struggle to detect new fraud patterns
- Rely heavily on manual review
- Are prone to high false-positive rates
With the growing volume of digital transactions, this approach creates bottlenecks and often delays decision-making.
Machine Learning: A Smarter, Real-Time Approach
Machine learning enables banks to go beyond rigid rule-based systems by allowing algorithms to learn from data and improve continuously. Here’s how ML transforms risk assessment:
- Predictive Analytics: ML algorithms analyze customer behavior to detect early signs of credit risk or default.
- Real-Time Fraud Detection: Models identify suspicious transaction patterns instantly.
- Customer Segmentation: ML groups customers based on risk factors, enabling personalized credit offerings.
- Cyber Threat Detection: ML tools detect anomalies in login attempts, network access, or unusual banking activities.
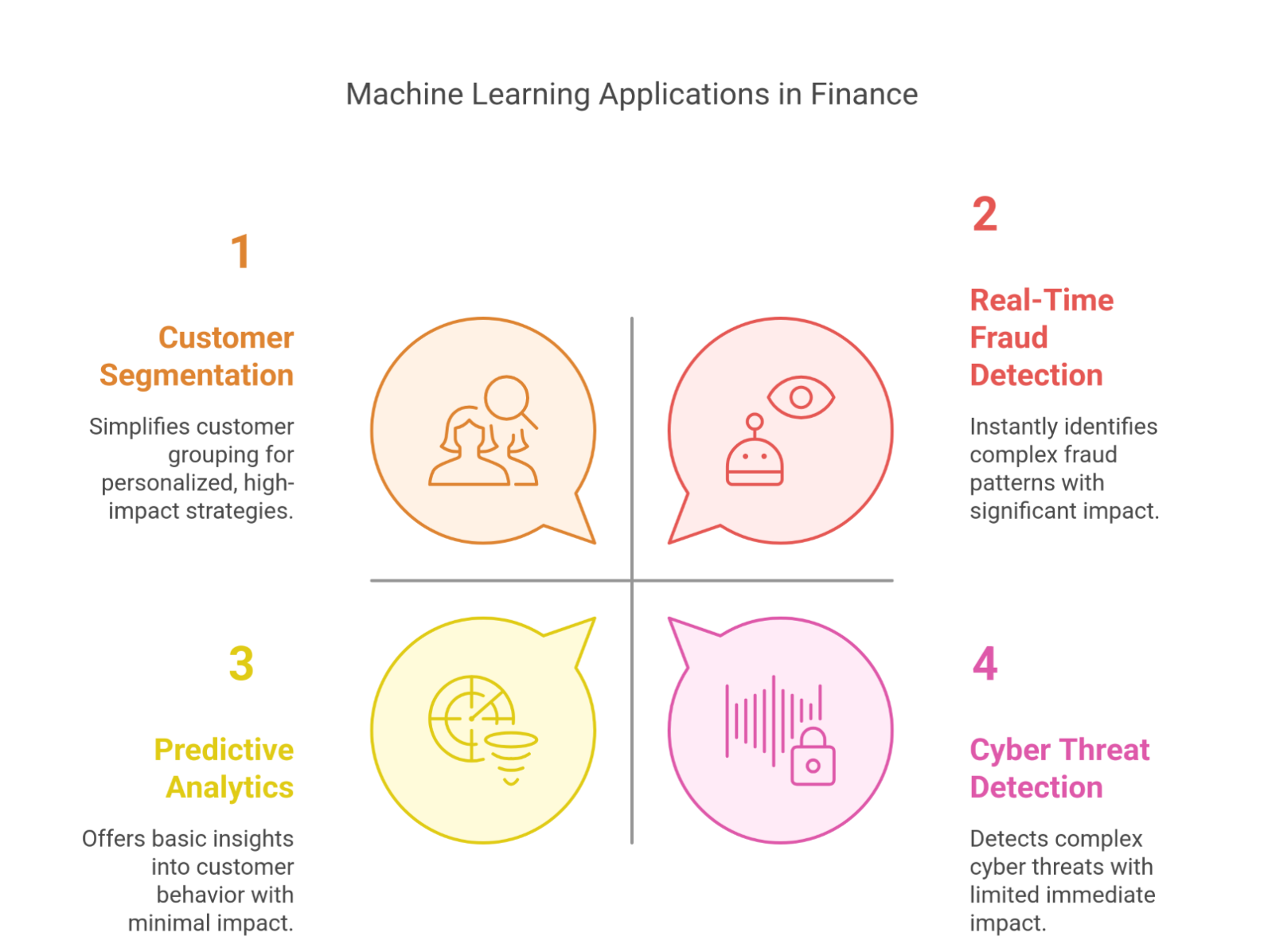
These capabilities significantly improve operational efficiency, enhance customer trust, and reduce financial loss.
Real-Life Examples of ML in Digital Banking Risk Assessment
1. How HSBC Utilizes Machine Learning in Financial Crime Prevention
HSBC implemented machine learning to detect suspicious activity within millions of transactions. By partnering with Quantexa, HSBC reduced investigation time by 20% while increasing the accuracy of AML alerts.
Source: Quantexa – HSBC Case Study
2. JP Morgan Chase – COiN Platform
JP Morgan’s COiN platform uses natural language processing (NLP) to assess legal and financial risk in loan documents. It analyzes 12,000 documents in seconds, a task that would take lawyers over 360,000 hours annually.
Source: JP Morgan COiN Overview
3. ICICI Bank – AI-Powered Fraud Detection
Closer to home, ICICI Bank in India integrated AI and ML into its digital banking services to flag high-risk transactions in real-time. This has improved fraud prevention by nearly 50% in online transactions.
Source: AI in Banking
The Role of AI in Custom Credit Scoring
Machine learning models can access alternate data points such as mobile usage patterns, utility bill payments, and social behavior (with consent). This is especially useful in countries like India, where many potential borrowers are underbanked.
Fintech players like KreditBee and CASHe are already using ML-based scoring to provide loans to first-time borrowers. This approach reduces non-performing assets (NPAs) and increases credit access.
Why It Matters for Ahmedabad’s Fintech and Banking Ecosystem
Ahmedabad is fast becoming a growing hub for digital innovation in fintech and banking. As regional banks and startups adopt intelligent financial products, the demand for custom machine learning solutions is growing too.
That’s where an AI development company in Ahmedabad like ours becomes crucial. From building fraud detection models to real-time credit scoring systems, our AI-powered solutions empower financial institutions to stay ahead of risk in a digital-first economy.
Ethical Considerations in ML-Driven Risk Assessment
While ML offers many benefits, financial institutions must ensure fairness, transparency, and accountability in AI models. Bias in training data can lead to unfair credit decisions, and explainable AI (XAI) is becoming essential to comply with financial regulations and maintain trust.
Future of Machine Learning in Digital Banking
Digital banking has only begun tapping into the potential of machine learning. Upcoming innovations include:
- Federated Learning: Privacy-preserving models trained across multiple devices without sharing sensitive data
- Self-Learning Models: Risk systems that adapt without human intervention
- Voice-Based Biometric Risk Scoring: Assessing risk through emotion and tone analysis in customer support calls
As banks embrace a digital-first model, machine learning will be at the heart of every major risk management strategy.
Build Smarter Financial Solutions with Theta Technolabs
We at Theta Technolabs craft tailored technology services for the evolving needs of the fintech and banking sector. Whether you're looking for mobile application development, web application development, or robust cloud infrastructure and setup, our team can help you leverage the power of machine learning and AI.
We’re more than just a service provider—we’re your strategic partner for growth in the digital financial space.
Get in touch with us at sales@thetatechnolabs.com to discuss your project.
Final Note
As an AI development company in Ahmedabad, Theta Technolabs is proud to support businesses in unlocking the potential of intelligent risk assessment. With the right tech stack and data strategy, digital banks can create more secure, inclusive, and future-ready financial ecosystems.